The case for decentralized compute in AI

The case for decentralized compute in AI
Leveraging unused resources and enhanced accessibility positions decentralized compute as a aggressive different in AI trend.
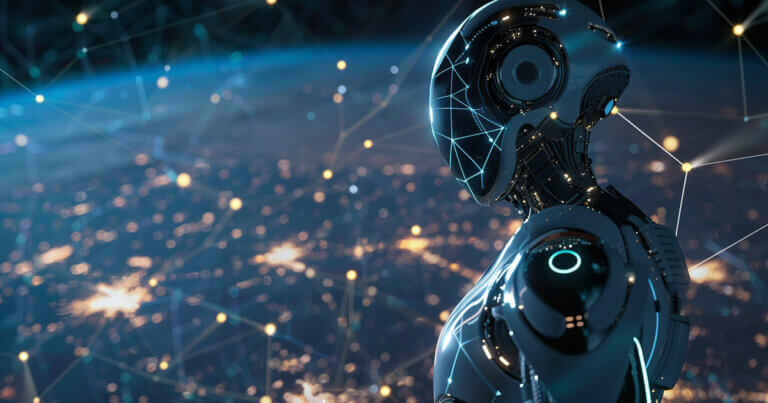
Mask art/illustration by strategy of CryptoSlate. Image includes blended remark which might well moreover encompass AI-generated remark.
The next is a visitor post by Jiahao Solar, CEO & Founder of FLock.io.
Within the ever-evolving panorama of synthetic intelligence (AI), the debate between centralized and decentralized computing is intensifying. Centralized companies love Amazon Web Companies and products (AWS) grasp dominated the market, offering sturdy and scalable suggestions for AI mannequin practicing and deployment. On the opposite hand, decentralized computing is emerging as a formidable competitor, presenting uncommon advantages and challenges that might well moreover redefine how AI models are professional and deployed globally.
Designate Efficiency through Unused Resources
One of the dear most well-known advantages of decentralized computing in AI is cost efficiency. Centralized companies invest carefully in infrastructure, declaring big knowledge companies and products with devoted GPUs for AI computations. This mannequin, whereas great, is costly. Decentralized computing, on the diversified hand, leverages “unused” GPUs from a range of sources across the area.
These is more likely to be personal computer programs, sluggish servers, and even gaming consoles. By tapping into this pool of underutilized resources, decentralized platforms can provide computing vitality at a section of the worth of centralized companies. This democratization of compute resources makes AI trend more accessible to smaller businesses and startups, fostering innovation and competition in the AI dwelling.
Enhanced Accessibility of GPUs
The area shortage of GPUs has vastly impacted the flexibility of slight businesses to stable the well-known computational vitality from centralized companies. Tall companies in general lock in long-time interval contracts, monopolizing entry to these severe resources.
Decentralized compute networks alleviate this dispute by sourcing GPUs from a various array of contributors, including particular particular person PC gamers and slight-scale companies. This elevated accessibility ensures that even smaller entities can fabricate the computational vitality they need with out being overshadowed by alternate giants.
Data Privateness and Client Preserve an eye on
Data privateness stays a paramount predicament in AI trend. Centralized programs require knowledge to be transferred to and saved within their infrastructures, successfully relinquishing particular person alter. This centralization poses well-known privateness risks. Decentralized computing supplies a compelling different by keeping computations end to the actual person. This will likely be carried out through federated studying, the set the records stays on the actual person’s instrument, or by utilizing stable decentralized compute companies.
Appleâs Private Cloud Compute exemplifies this vogue by integrating several iCloud compute nodes around a particular particular person, thereby declaring knowledge privateness whereas leveraging cloud computational vitality. Even supposing this kind aloof entails a level of centralization, it underscores a shift in the direction of larger particular person alter over knowledge.
Verification Protocols and Security
No subject its advantages, decentralized computing faces several challenges. One severe dispute is verifying the integrity and security of decentralized compute nodes. Guaranteeing that these nodes are no longer compromised and that they provide precise computational vitality is a complex dispute.
Advances in blockchain technology provide possible suggestions, enabling self-proofing mechanisms that test the legitimacy of compute nodes with out compromising security.
Preserving Data Privateness in Decentralized Systems
One other well-known dispute is the skill exposure of non-public knowledge for the length of decentralized computations. AI models thrive on big datasets, however with out privateness-keeping applied sciences, decentralized practicing might well moreover menace knowledge breaches. Tactics equivalent to Federated Finding out, Zero-Data Proofs, and Entirely Homomorphic Encryption can mitigate these risks.
Federated Finding out, broadly adopted by valuable companies since 2017, lets in knowledge to remain local whereas aloof contributing to mannequin practicing. By integrating these encryption and privateness-keeping applied sciences into decentralized compute networks, we can pork up knowledge security and particular person privateness, pushing the boundaries of what decentralized AI can attain.
Bandwidth and Efficiency Concerns
The efficiency of decentralized compute networks is another dwelling of predicament. The transmission efficiency in a decentralized plan will inevitably mosey in the motivate of centralized clusters as a result of allotted nature of the community. Historical anecdotes, equivalent to AWS transferring knowledge from Toronto to Vancouver for the length of a blizzard, highlight the logistical challenges of info transmission.
On the opposite hand, trends in AI suggestions love LoRA splendid-tuning and mannequin compression can motivate mitigate these bandwidth bottlenecks. By optimizing the records switch processes and refining mannequin practicing suggestions, decentralized compute networks can attain efficiency levels which are aggressive with their centralized counterparts.
Bridging the Gap with Rising Technologies
The blending of blockchain technology with AI supplies a promising avenue for addressing quite loads of the challenges faced by decentralized computing. Blockchain supplies a transparent and immutable ledger for monitoring knowledge provenance and compute node integrity. This ensures that all members in the community can belief the records and computations being performed.
Additionally, blockchain’s consensus mechanisms can facilitate decentralized governance, enabling communities to collectively arrange and toughen the community.
Moreover, trends in Federated Finding out and Homomorphic Encryption are pivotal in making certain that knowledge privateness is maintained whereas leveraging the allotted nature of decentralized compute networks. These applied sciences enable AI models to learn from allotted datasets with out exposing sensitive knowledge, thereby balancing the need for big quantities of info with stringent privateness requirements.
The Plot forward for Decentralized Compute in AI
The skill of decentralized compute networks to revolutionize AI trend is ample. By democratizing entry to computational resources, enhancing knowledge privateness, and leveraging emerging applied sciences, decentralized AI can provide a sturdy different to centralized programs. On the opposite hand, the slump is fraught with challenges that require innovative suggestions and collaborative efforts from the AI and blockchain communities.
As we switch forward, we must continue exploring and growing decentralized computing suggestions that handle these challenges. By fostering a collaborative ecosystem, we can fabricate definite that that the benefits of AI are accessible to all, promoting a more equitable and innovative future for AI trend.
Source credit : cryptoslate.com